Change is underway in the ESG sector, and it is being accelerated by the growing role ESG data is playing across the professional investment community. This evolution is creating compelling technology driven opportunities for savvy institutional investors, analysts and ESG advisors to leverage ESG data to improve their investment outcomes, manage risk and advise clients. If you involved in this ecosystem, it’s well worth your time to investigate the latest developments in this space. We delve into the opportunities below.
The standard approach to ESG research
For starters, it’s now well understood in the institutional investment world that utilizing high quality ESG data is a powerful tool to better mitigate against corporate risk. Demand for ESG data is booming as a result.
Most professional investors access their ESG data and analysis from organisations such as MSCI and Sustainalytics. These data providers typically cover large-cap companies in the world. Below is their analysts based ESG summaries for Apple Inc (AAPL).
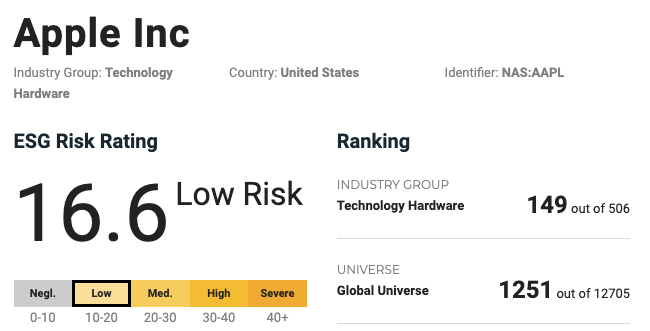
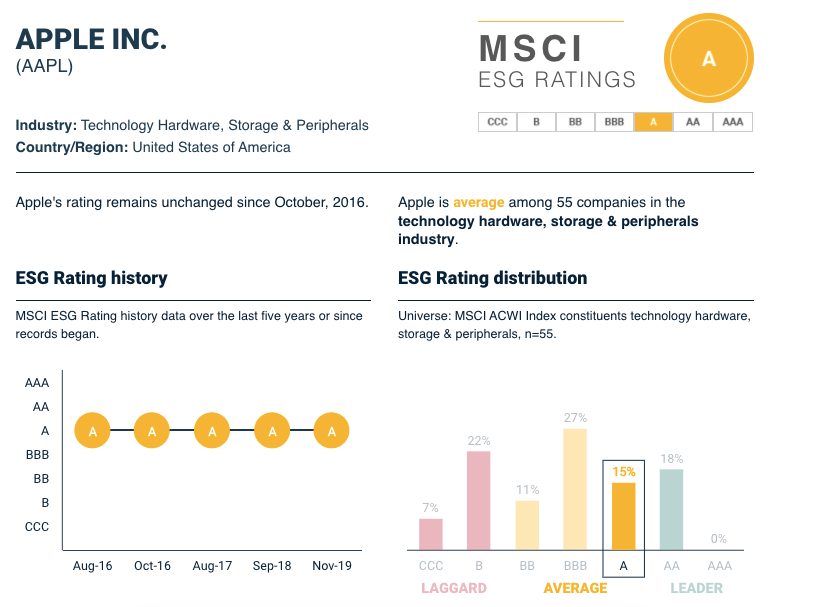
Both Sustainalytics and MSCI do a great job of delving deep into company disclosures to provide a picture of how each company is performing on ESG issues. Their analysts have a well-trodden process of sifting through corporate disclosures with a view to providing a ESG risk rating and analysis.
Is this approach working?
What if this analyst-driven ESG research which fund managers are relying upon is not as comprehensive and up-to-date as they think it is?
There’s a lot to unpack here. Firstly, it’s worth bearing in mind that larger companies have a much stronger track record in ESG disclosure and performance than smaller companies. As shown below, the ESG score gap is significant between large caps and small / mid caps.

Whilst the correlation between ESG disclosure and ESG performance is high (for the simple reasons that companies which are doing good things tend to sing about it, larger companies have more analyst coverage and higher abilities to report administrative-heavy ESG data. It’s important to understand that analyst created research is dependent upon company created disclosures. So there’s a strong correlation between the quality of the ESG research and the quality of the disclosure. This is by design, and existing data providers aim to standardize reported data in a way that can be used for comparisons, not focusing on predictive power.
If a company is providing selective ‘greenwash’ type ESG disclosures which are focused on marketing outcomes rather than real ESG factors, then the quality of the ESG research will be low. It becomes a case of garbage in, garbage out. And equally, if a smaller company doesn’t have the resources to provide even the most basic ESG disclosures, there’s not much an ESG analyst can do to create a meaningful ESG report. Both situations are common challenges which create significant issues with the standard approach of relying upon analyst created ESG research.
It’s important to understand that analyst created research is dependent upon company created disclosures. So there’s a strong correlation between the quality of the ESG research and the quality of the disclosure. This is by design, and existing data providers aim to standardize reported data in a way that can be used for comparisons, not focusing on predictive power.
There are other significant issues to be aware of. The timing of company disclosures is a big one. Most companies compile ESG related reports once a year which may take them a few months to complete. By the time they publish their ESG report, the information could be six months out of date. So when ESG analysts are producing their research, they are creating an ESG picture of the company from the past. This time lag, combined with a lack of framework or report standardization creates significant information gaps in the standard ESG research process.
Finally, it’s worth understanding what an ESG analyst has to work with. If you google ‘Apple ESG’ for example, your search results in over 500,000 pieces of media. Most analysts have time to read through a handful of articles at most when they complete an ESG report. That means there are giant chasm between the information going into the analyst ESG reports and the potential information which could be used. Bearing in mind the world is awash with unstructured data, with some estimates suggesting 90% of global data was created in the last two years alone, this information gap is massive. And as most fund managers will agree, information gaps mean money.
A new normal emerging
Professional investors have long demanded research which adds value, and the same is true in the world of ESG research. With the above-mentioned challenges in mind, demand has been growing rapidly for ESG research which is objective, comprehensive and up-to-date.
The good news is this gap is being addressed by AI derived ESG data which doesn’t depend on analyst input and potential biases. As shown below, AI-driven ESG research offers an objective and comprehensive approach that complements traditional analyst approaches to truly give you the full picture.

ESG Analytics: An AI leader in the making
ESG Analytics is one of the new breed of ESG data providers aiming to create the value the institutional investment world wants and needs. ESG Analytics’ AI technology scans the world of unstructured media to understand other things about companies, flags analysts may have missed, or issues which have happened more recently than the information analysts are relying upon. As shown below, the ESG Analytics scanning process is thorough.
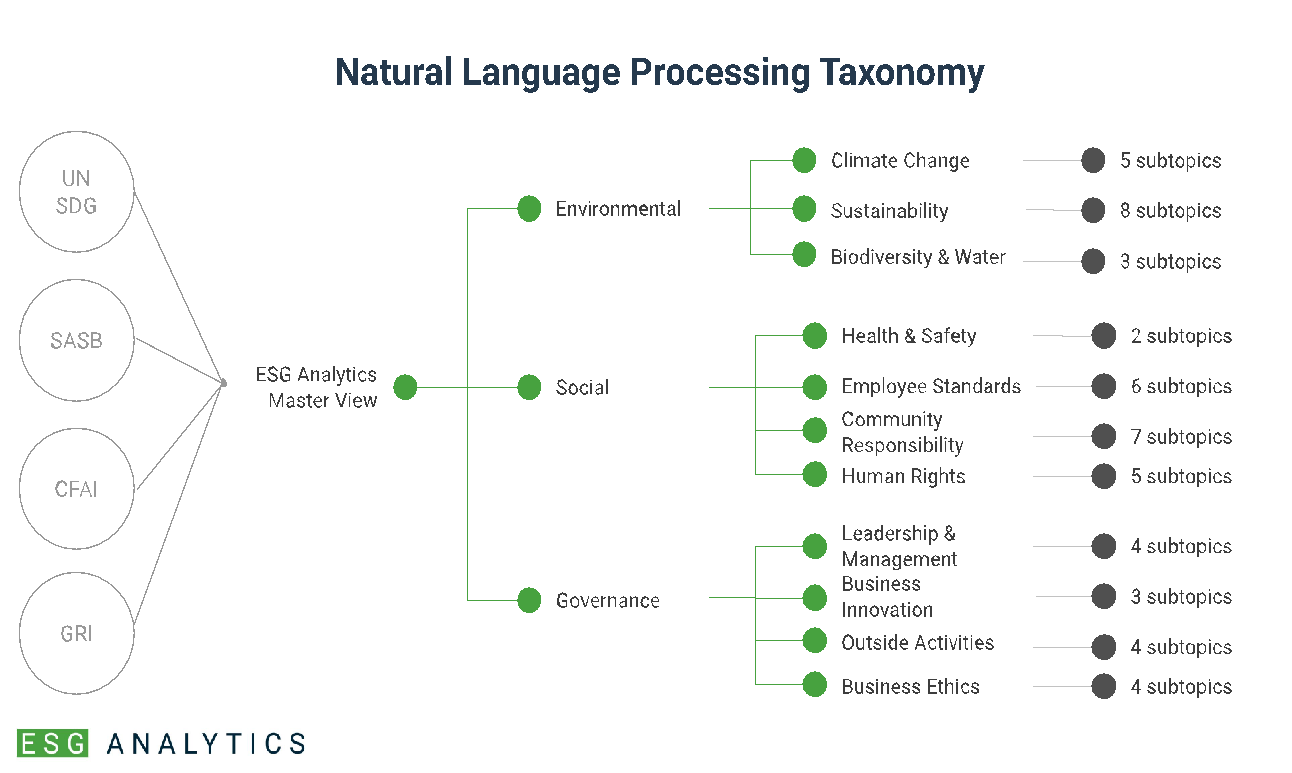
This AI-focused approach creates a master taxonomy of the ESG world based on ESG frameworks and knowledge, which is used to create powerful ESG algorithms that capture and create high value-add data for investors. Importantly, this is data incorporates not only what companies say about themselves, but also what the rest of the world says about the companies. It’s objective data, and thus it’s particularly valuable as an investment tool, particularly in combination with the subjective analyst research.
Importantly, this is data incorporates not only what companies say about themselves, but also what the rest of the world says about the companies.
Example: Apple
Let’s circle back to Apple as example which shows the difference between the old analyst and new AI approaches to ESG research.
Sustainalytics has a current ESG rating on Apple of Low Risk. However, ESG Analytics’ all-encompassing AI data analysis has additional, more granular insights that can help us complete our analysis.
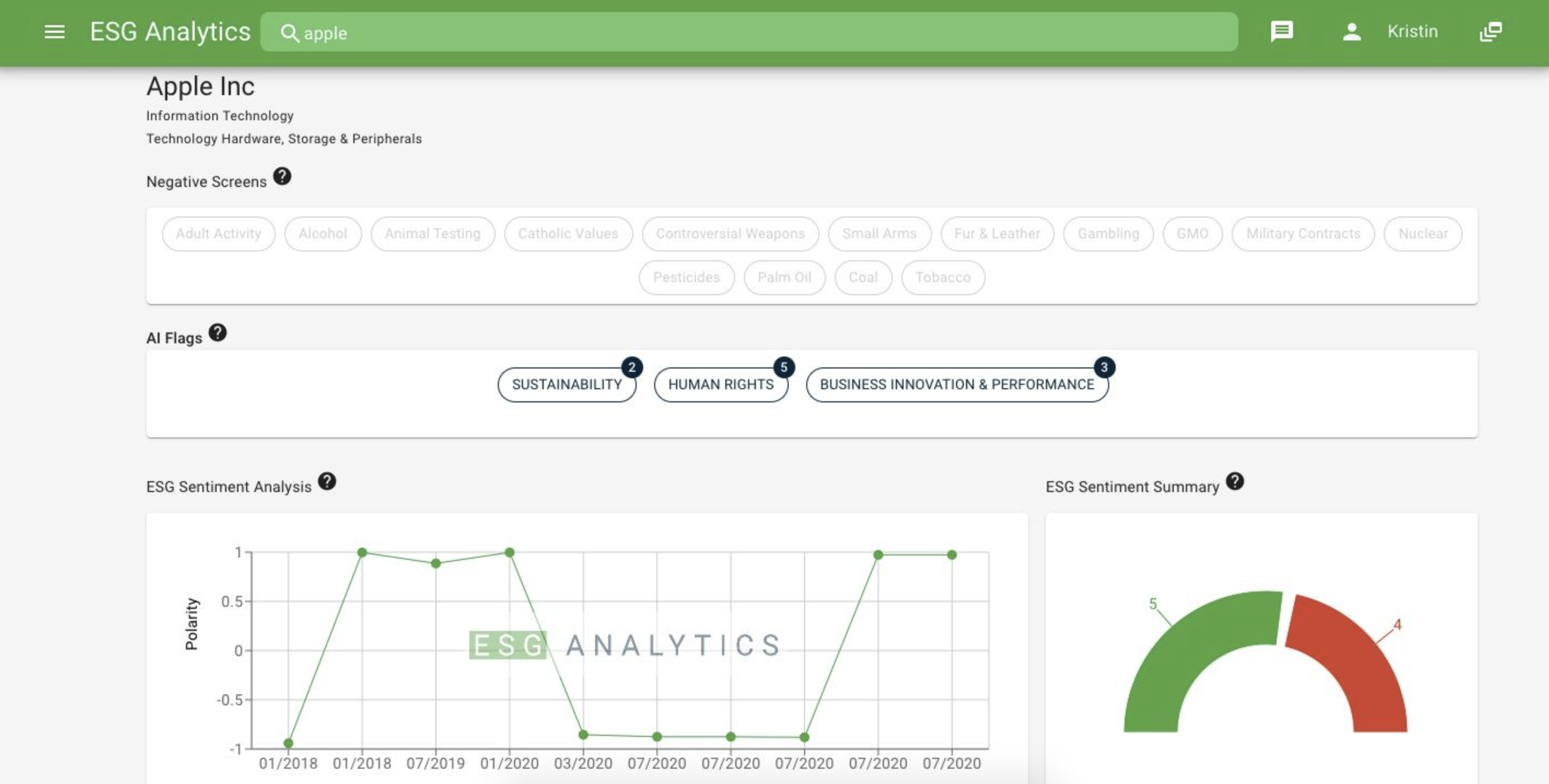
By the time a flag/topic is entered into ESG Analytics - it has passed through a series of algorithms which can understand media with the brain of an ESG Analyst. Whats left is data that has a material impact on a company while ignoring the thousands of irrelevant data points. Effectively uncovering hidden risk and opportunity in the world around us.
Let's examine the components individually. Currently highlighting 3 categories of ESG flags for Apple which are not showing up in the analyst research. Here are a few examples:
- Human Rights – The US State Department is warning a number of US companies including Apple that China’s Xinjiang has forced labour issues. Apple has sourced staff uniforms from Xinjiang in the past, so this potential red flag may indicate Apple’s current or past strategy to protect human rights needs work. If we hover over the ESG chart, we can see specifically identified sources of material ESG risk (and opportunities) that we can investigate further.
If we dive into one of these flags, we can actually pull up the sources that we're identified with the ESG AI and see intelligently highlighted components for quick analysis. This was classified as extremely negative by the additional sentiment indicators (polarity of -0.8758) - more on this later.

- Sustainability – Apple is aiming to remove carbon from its supply chain and products by 2030. This is a positive flag because ESG friendly companies are generally more proactive on this measure.
- Business Innovation – It was recently announced that of the record 333,530 US patents granted in 2019, IBM and Samsung were the leaders whilst Apple performed less favourably. This is a potential red flag as it may highlight that Apple’s track record as an innovation leader may be unsustainable.
By the time a flag/topic is entered into ESG Analytics - it has passed through a series of algorithms which can understand media with the brain of an ESG Analyst. Whats left is data that has a material impact on a company while ignoring the thousands of irrelevant data points. Effectively uncovering hidden risk and opportunity in the world around us.
Arguably, these three data points which didn’t surface in the analyst ESG research on Apple, add significant value in combination with the analyst research and only represents a fraction of what was picked up. Interpreting the traditional and AI analyses in combination may allow investors to understand that when Apple is controlling the dialogue, its ESG performance looks relatively good. However, from a more objective perspective, the company may have more work to do, and importantly, it may face greater risks than some investors believe.
ESG Analytics’ data can also be used to look identify the sentiment of material ESG topics. Here’s the Apple data.

This data shows that Apple’s ESG topic exposure has been roughly 50/50 positive/negative since January 2018, and has been a volatile input. 50/50 is a very different performance to the ‘Low Risk’ ESG ratings presented by the leading analyst led organisations. It’s the difference between great and mediocre, and it’s valuable information for investors as it highlights that Apple’s ESG risks may be higher than the market realises at present - which drastically increases the potential for future headline risk and longer term portfolio impact. With this technology, we can actually chart the material ESG factors of a company over time.
Considering Apple is one of the world’s largest companies, and yet there’s still a glaring ESG data gap at play, it highlights how big an opportunity AI derived ESG data is addressing. Of the 60,000 companies across 64 exchanges and 40 countries which ESG Analytics covers, most have little or no disclosure based ESG data available. That’s a lot of gaps to cover. And it’s a testament to the power of data which is where the future lies in the ESG world.
Of the 60,000 companies across 64 exchanges and 40 countries which ESG Analytics covers, most have little or no disclosure based ESG data available. That’s a lot of gaps to cover. And it’s a testament to the power of data which is where the future lies in the ESG world.
Conclusion
The professional investment world at large now has access to value-adding data of a quality which was only available to the world’s top performing hedge fund managers in the past. As illustrated above, AI sourced ESG data is particularly useful in conjunction with analyst sourced ESG research. It addresses the significant information and timing gaps prevalent in the ESG sector, and allows investors to weight the analyst research within a context of a wider world view.
ESG Analytics is emerging as a key provider of high quality AI derived ESG data.
We would love to show you what we are working on at ESG Analytics, you can use this link to find a time that works for us both.